Data Annotation: The Key to Unlocking AI Excellence
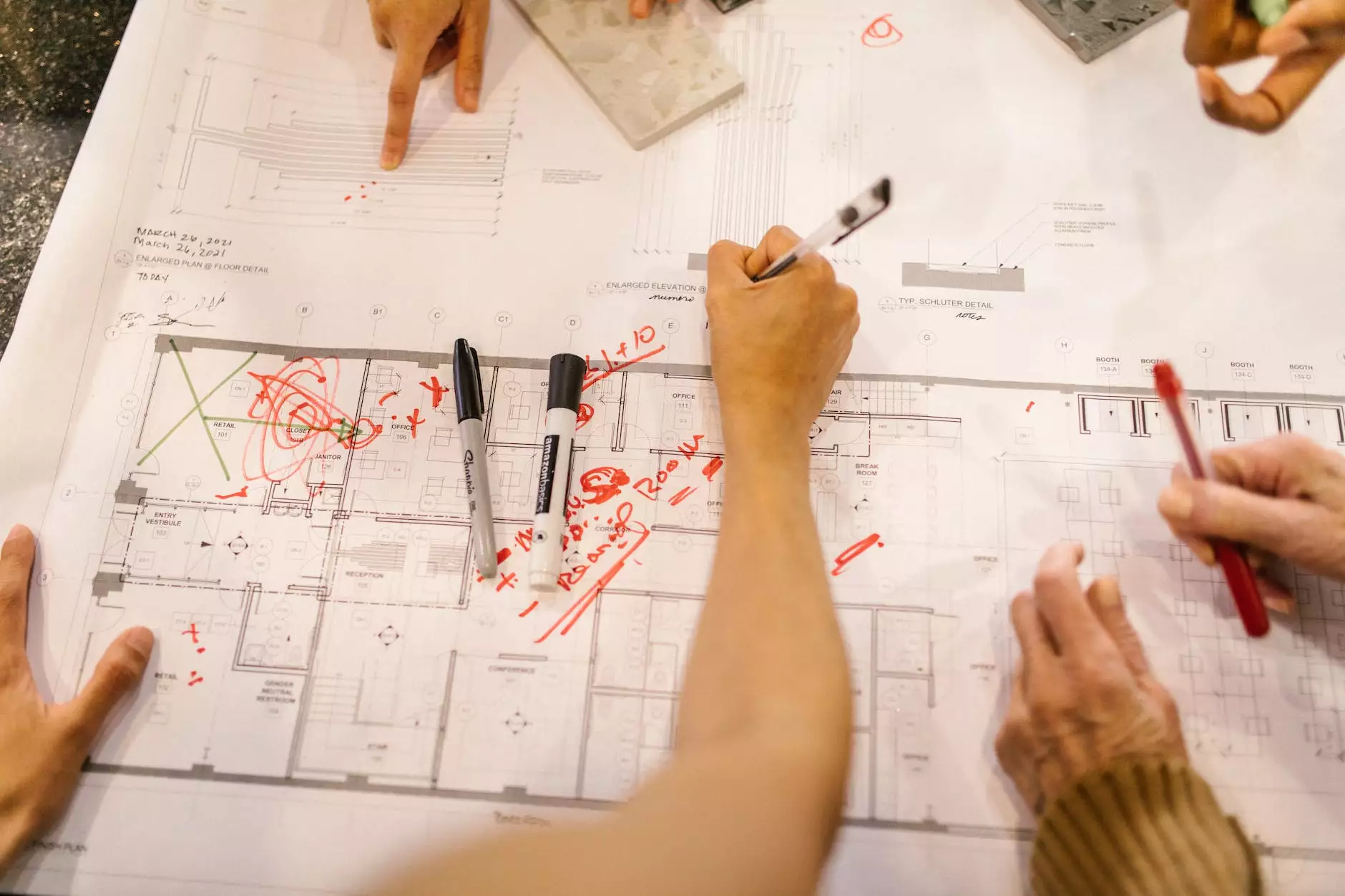
Data annotation has emerged as an essential process in the development of artificial intelligence (AI) and machine learning (ML). With the explosion of data in today’s world, the need for accurate and effective data labeling has never been more critical. Companies like keylabs.ai specialize in providing robust data annotation tools and platforms that empower businesses to harness the full potential of AI. This comprehensive guide explores the intricacies of data annotation, its importance in various industries, and how it can drive business success.
Understanding Data Annotation
At its core, data annotation involves the process of labeling data to train machine learning models. This includes tagging images, transcribing audio, and identifying relevant features in text data. By accurately labeling datasets, businesses can train algorithms to recognize patterns and make predictions based on new, unseen data. This process is crucial for various applications, including but not limited to:
- Image Recognition: Labeling images for object detection and classification.
- Natural Language Processing: Annotating text data for sentiment analysis, entity recognition, and language translation.
- Audio Processing: Transcribing and labeling audio files for speech recognition and analysis.
The Importance of Data Annotation in AI Development
Data is often referred to as the new oil, and just like oil, it needs to be refined to be useful. In the context of AI, the "refinement" process is data annotation. Accurate and high-quality labeled data is essential for developing robust AI applications. Below are several reasons why data annotation is paramount:
1. Enhancing Machine Learning Accuracy
The performance of machine learning models heavily depends on the quality of the training data. Annotated datasets help models learn with greater precision, leading to more accurate predictions and insights. Without proper annotation, models may produce biased results, misclassifications, or inaccuracies.
2. Facilitating Diverse Applications
Data annotation enables a wide variety of applications across diverse industries. From healthcare to finance, businesses leverage annotated data to develop sophisticated algorithms that improve operations, personalize customer experiences, and optimize decision-making processes.
3. Optimizing Resource Allocation
By implementing efficient data annotation processes, companies can better allocate their resources. Annotated datasets lead to faster development cycles, shortening the time-to-market for AI applications. This can significantly enhance a company's competitive edge in an increasingly crowded marketplace.
4. Building Consumer Trust
For businesses that prioritize consumer trust and experience, data annotation plays a vital role. By ensuring that algorithms are trained on accurate datasets, businesses can enhance their transparency. This trust is essential in industries like finance and healthcare, where user data is sensitive and must be handled with the utmost care.
Types of Data Annotation Techniques
There are several types of data annotation techniques, each tailored to different data types and use cases. Let’s explore the most common methods:
1. Image Annotation
This involves labeling images to help AI systems understand and interpret visual data. Common techniques include:
- Bounding Boxes: Drawing boxes around objects of interest in an image.
- Semantic Segmentation: Classifying each pixel in an image to understand the context.
- Keypoint Annotation: Marking significant points (joints, features) on objects.
2. Text Annotation
Text annotation involves labeling segments of text for various purposes, including:
- Entity Recognition: Identifying and labeling entities such as names, dates, and locations.
- Sentiment Analysis: Classifying text as positive, negative, or neutral.
- Text Classification: Categorizing text documents based on predefined labels.
3. Audio Annotation
Audio annotation is crucial for training speech recognition models. Techniques include:
- Transcription: Converting spoken language into text.
- Speaker Identification: Labeling who is speaking in a multi-speaker audio clip.
- Intent Detection: Analyzing the intent behind spoken phrases or commands.
Choosing the Right Data Annotation Tool or Platform
With numerous data annotation tools and platforms available, selecting the right one can be overwhelming. Here are some critical factors to consider when choosing a data annotation tool or platform:
1. Ease of Use
The interface of the tool should be intuitive and user-friendly. An easy-to-navigate platform will enhance productivity and reduce training time for employees.
2. Scalability
As your data needs grow, your annotation tool must be able to scale accordingly. Choose a platform that can handle large datasets and increase its functionality as your company expands.
3. Support for Various Data Types
Consider whether the tool supports multiple data types (text, images, and audio). A versatile platform will enable you to handle various projects and maintain a consistent workflow.
4. Quality Assurance Features
Quality assurance is vital for producing high-quality annotated datasets. Look for tools that provide review mechanisms, allowing for double-checking of annotations and ensuring accuracy.
5. Cost-effectiveness
Evaluate the pricing structure of different platforms. Ensure that the investment aligns with the benefits and features offered.
How Keylabs.ai Elevates Data Annotation
keylabs.ai stands out in the data annotation landscape by offering a comprehensive platform designed for various industries. Here are some ways in which Keylabs.ai enhances data annotation for its users:
1. Advanced Annotation Techniques
Keylabs.ai utilizes cutting-edge technology to deliver advanced annotation techniques, including automated labeling and AI-assisted workflows. This dramatically reduces the time required for manual annotation without sacrificing quality.
2. Superior Data Quality Assurance
The platform integrates quality assurance protocols that ensure accuracy and reliability in data annotation. This is particularly crucial for businesses relying on precision, such as in health tech and autonomous driving.
3. Flexible Integration Capabilities
Keylabs.ai's data annotation platform seamlessly integrates with existing systems, allowing businesses to streamline their workflows and optimize productivity without disruptive changes to their current processes.
4. Dedicated Support and Customization
With a dedicated support team and customizable solutions, keylabs.ai works closely with clients to tailor the annotation process to suit their specific needs and goals. This personalized approach optimizes outcomes and adds value to businesses.
Conclusion: Embrace the Power of Data Annotation
In a data-driven world, embracing data annotation is critical for any business seeking to excel in AI and machine learning. By investing in quality annotated datasets, organizations can enhance model performance, improve decision-making, and ultimately drive business growth. With industry leaders like keylabs.ai, companies have access to the necessary tools and platforms to succeed in this arena.
As AI continues to evolve, the importance of high-quality data annotation will only increase. The future of successful AI development rests on how effectively businesses adapt and utilize these annotation processes. Embrace the power of data annotation and unlock your organization's potential today!