Mastering Image Annotation for Machine Learning: A Comprehensive Guide
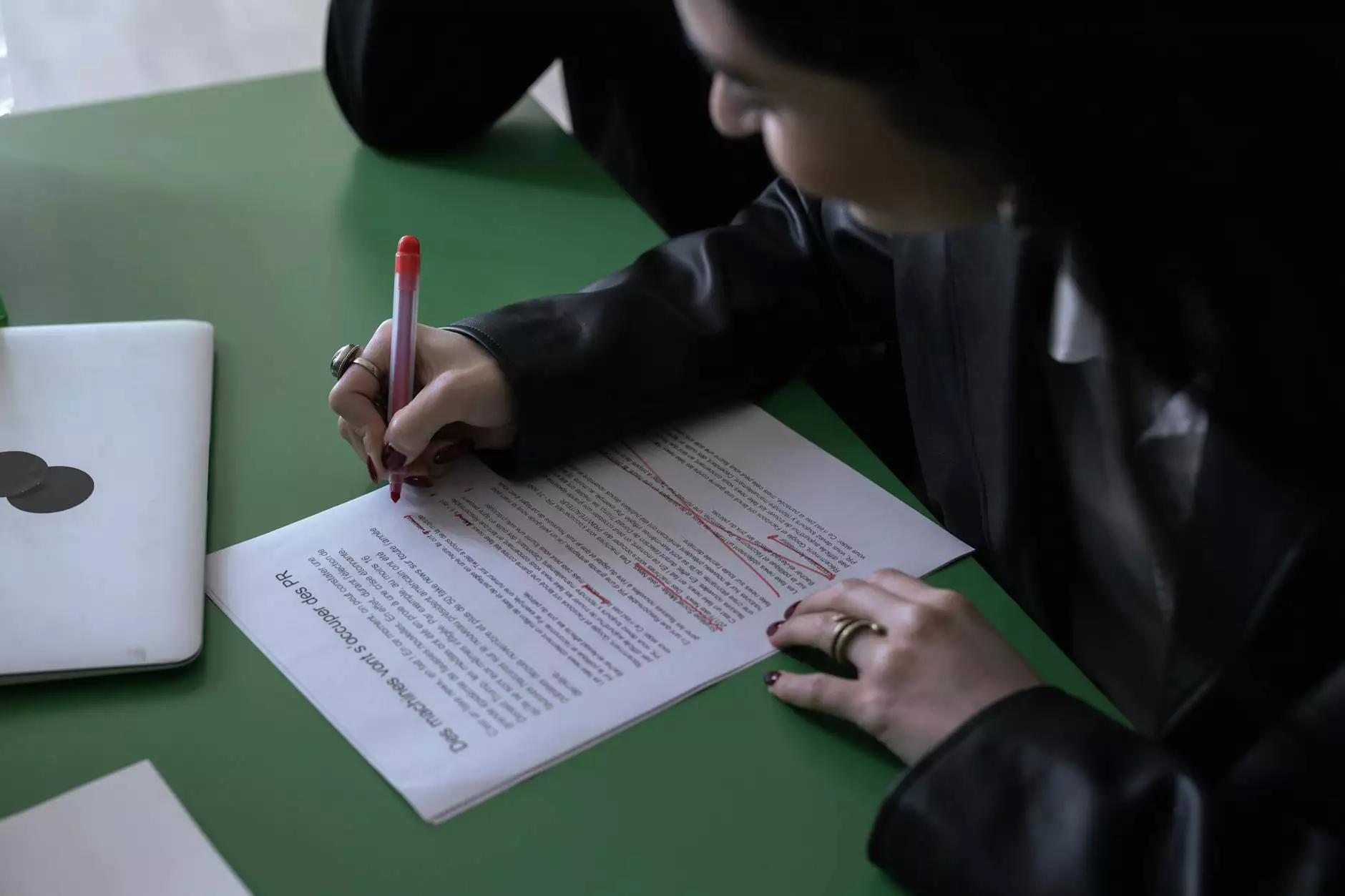
In the rapidly evolving landscape of technology, image annotation for machine learning has emerged as a cornerstone technique that stratifies the capabilities of artificial intelligence (AI). As data becomes more abundant and machine learning models become increasingly complex, the need for precise and well-structured data—particularly image data—can't be overstated. This article delves into the essentials of image annotation, its importance in machine learning, the various techniques involved, and how businesses can leverage these strategies to enhance their services.
Understanding Image Annotation
At its core, image annotation refers to the process of labeling or tagging images with predefined values. This process creates datasets that machine learning algorithms can use to learn and make predictions. Imagine training a model to recognize various objects in photographs; the model needs prior knowledge to identify these objects accurately. That's where image annotation comes in.
The Importance of Image Annotation in Machine Learning
Image annotation is critical in various artificial intelligence applications, including:
- Object Detection: Teaching models to identify and categorize objects within images.
- Image Segmentation: Dividing images into multiple segments to analyze and process each part individually.
- Facial Recognition: Enabling systems to identify and verify human faces in images.
- Self-Driving Technology: Assisting autonomous vehicles in interpreting their environments accurately.
The Process of Image Annotation
The process of image annotation for machine learning involves several key steps:
1. Data Collection
The first step is to gather a comprehensive dataset of relevant images. This dataset should encompass a diverse range of scenarios to ensure the machine learning model can generalize well to unseen data.
2. Choosing the Annotation Type
Depending on the use case, businesses can choose different annotation types:
- Bounding Boxes: Rectangular boxes that outline specific objects.
- Polygon Annotation: More precise than bounding boxes, used to identify irregularly shaped objects.
- Landmark Annotation: Specific points marked on images, useful in facial recognition or anatomical studies.
- Semantic Segmentation: Classifying every pixel in an image, ideal for tasks requiring detailed recognition.
3. Annotation Tools
Numerous tools are available for image annotation, ranging from open-source options to high-end commercial products. Examples include:
- Labelbox: A user-friendly platform with robust features for managing datasets.
- RectLabel: A MacOS application that supports various annotation types.
- CVAT: An open-source tool developed by Intel for computer vision tasks.
4. Quality Control
Maintaining high standards of data quality is crucial. Implementing a quality control process helps to mitigate human errors that can arise during annotation. Some practices to enhance quality control include:
- Double Annotation: Having multiple annotators label the same image and then comparing results.
- Guidelines and Training: Developing comprehensive guides for annotators to follow ensures consistency.
- Review Cycles: Regularly reviewing annotations as part of a feedback loop can significantly improve quality.
The Impact of Accurate Image Annotation
Inaccurate annotations can lead to poor model performance, resulting in lost opportunities and wasted resources. Here’s how accuracy impacts various industries:
1. Healthcare
In the medical field, precise image annotation aids in diagnosing diseases, offering accurate assessments based on medical imaging techniques like X-rays or MRIs. Mis-labeling can lead to misdiagnosis, making it crucial for training algorithms used in healthcare applications.
2. Autonomous Vehicles
For self-driving cars, precise image annotation helps computers "see" and understand their surroundings. Accurate data for objects such as pedestrians, traffic signs, and other vehicles is essential for the safe operation of autonomous systems.
3. Retail and E-commerce
In retail, image annotation enhances customer experiences through improved object recognition in product images. Annotated images can be used to power search features, enabling customers to find items quickly and efficiently.
Challenges in Image Annotation
Despite its significance, image annotation for machine learning presents several challenges:
1. Data Privacy
As businesses collect image data, they must comply with data protection laws. Understanding the implications of data privacy regulations is crucial to avoid legal issues.
2. Scalability
As the demand for annotated images grows, scaling the annotation process without sacrificing quality becomes a significant challenge.
3. Complexity and Ambiguity
Sometimes, the interpretation of what an image depicts can be ambiguous. This subjectivity can lead to inconsistent annotations, which ultimately affects the model's training.
Conclusion: The Future of Image Annotation in Business
As the need for sophisticated machine learning models continues to escalate, the importance of image annotation for machine learning will only increase. Businesses across various sectors must invest in high-quality data annotation to stay competitive and leverage the power of AI.
Organizations like KeyMakr can provide exceptional insights into how to execute these processes efficiently in industries like Home Services, Keys & Locksmiths, greatly benefiting from innovations in machine learning.
Getting Started with Image Annotation
If your business is considering integrating machine learning into its operations, focusing on image annotation is a wise investment. Here are some tips to get started:
- Assess Your Needs: Determine what type of images you need to annotate and the goals of your machine learning project.
- Select the Right Tools: Choose annotation tools that fit your business model and workflow.
- Establish Clear Guidelines: Create thorough instructions for annotators to maintain consistency across the dataset.
- Iterate and Improve: Use feedback from the model's performance to tweak annotations as necessary, aiming for continuous improvement.
By embracing the power of image annotation for machine learning, businesses can unlock new capabilities, improve operational efficiencies, and foster innovation. As technology evolves, the leaders in this space will not only be those who adopt AI but those who understand and implement high-quality data annotation practices.